LAB-Bench: Measuring Capabilities of Language Models for Biology Research

Our goal at FutureHouse is to automate scientific research. Today, we are announcing a new set of evaluations for benchmarking language models and language agents which should help us measure progress towards that goal. We are calling our eval set the Language Agent Biology Benchmark, or LAB-Bench.
In total, we are releasing 2,457 questions across 8 broad categories.

Procedural Evaluations
Most evals for science today measure knowledge. By contrast, our evals are intended to measure whether AI models can perform the tasks needed to do scientific research. This includes extracting information from the scientific literature (LitQA2), retrieving information from databases (DbQA) and supplementary information (SuppQA), manipulating biological sequences (SeqQA), reasoning about scientific figures (FigQA) and tables (TableQA), and designing biological protocols (ProtocolQA). As their names suggest, the benchmarks are all presented in multiple-choice format, because for most of the categories we do not believe that models are reliable for automatic evaluation today. We believe that performing similarly-well to humans on all of these benchmarks is a necessary (but not sufficient) condition for an AI system to be valuable as a scientific collaborator or assistant for humans.
We also include, in particular, a set of 41 Cloning Scenarios, which are challenging multi-step questions that we expect would take a trained molecular biologist at least 10 minutes to answer completely. We believe that achieving high accuracy on the cloning scenarios is likely a sufficient condition for an AI system to add value as a scientific collaborator or assistant for human molecular biologists. Moreover, it is difficult to imagine that a useful AI assistant for molecular biology would be incapable of correctly answering the Cloning Scenarios, so performance on the Cloning Scenarios may in fact be necessary and sufficient for a valuable AI assistant for biology. The cloning scenarios may thus be useful as a benchmark for evaluating the potential of AI systems to augment (or, depending on your outlook, displace) human labor in science.
What are the results?
As we report in our paper, all available models today deeply underperform humans on all of the tasks we present here [1], with the exception of Claude 3.5 Sonnet, which narrowly exceeds humans on TableQA precision.
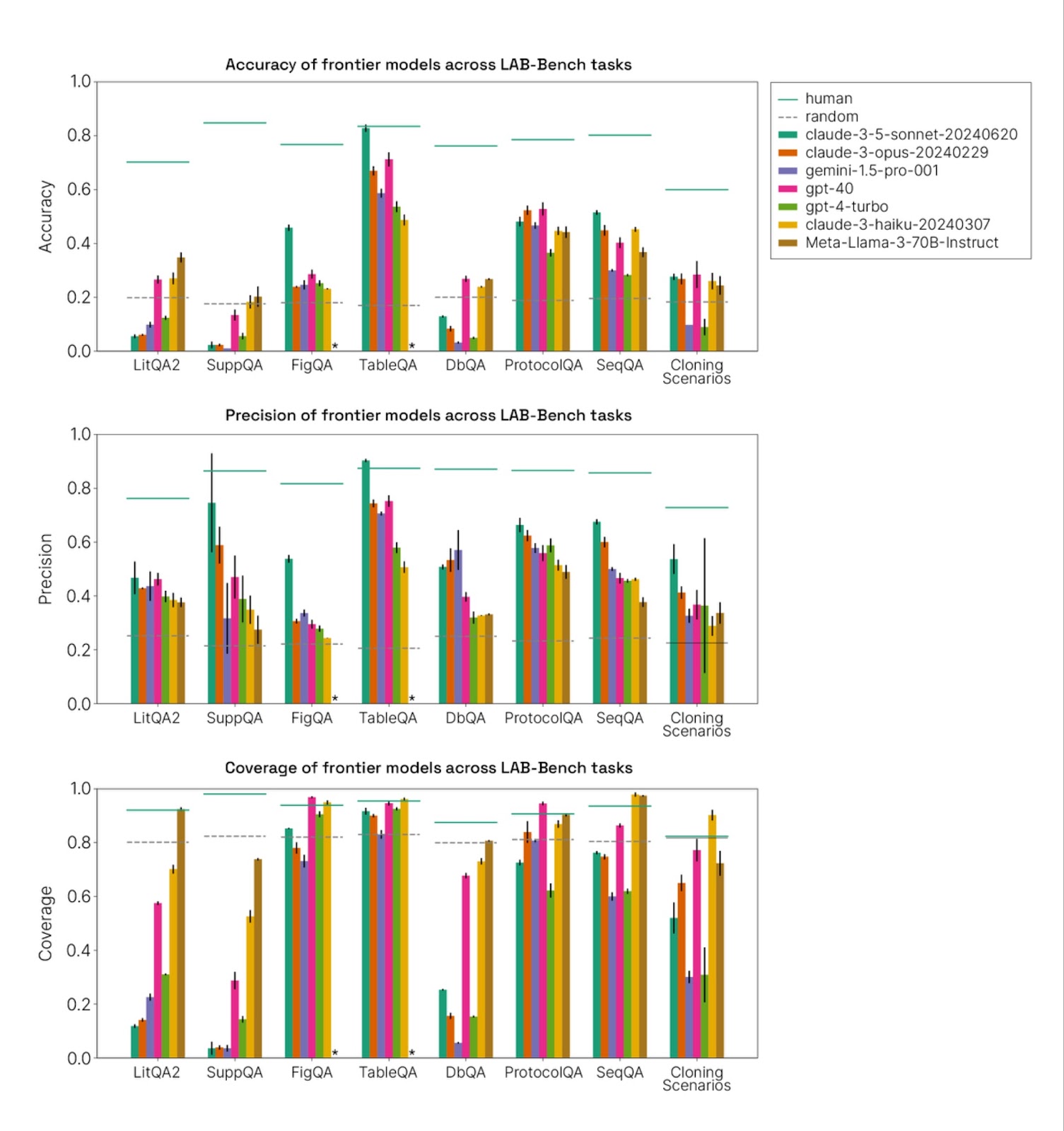
In some categories, including DbQA, SeqQA, LitQA2, and SuppQA, this is because many of the tasks require tools, and we do not evaluate the models with tools. (Humans, by contrast, were allowed to use any tools they had available, except AI-based chat tools) We anticipate that models augmented with the correct tools will achieve significantly increased performance on many of the subtasks in those categories and may meet or exceed humans in some cases. The poor performance of models in these categories reflects the need for additional infrastructure to perform many of the critical activities required for scientific research.
However, other categories such as FigQA, TableQA, and ProtocolQA, do not require tool use, and nonetheless see substantially sub-human performance by most models. In particular, we found that all models except Claude 3.5 Sonnet perform near chance on FigQA, a visual reasoning benchmark, suggesting major visual reasoning deficiencies in most models. FigQA requires significant attention to detail and is also often “multi-hop,” requiring the model to identify multiple elements within a single figure, which may explain its difficulty; however, humans achieve high performance on it. These benchmarks may be useful for model builders in the future, and improvements on them will be necessary for models to contribute significantly to science in the future.
Limitations:
Two limitations of this benchmark stand out in particular. Firstly, because questions are presented in multiple-choice format, models can sometimes achieve above random performance without actually knowing how to deduce the answer to a question, by eliminating unlikely distractors and guessing. This is particularly evident on the Cloning Scenarios, where some models perform above chance on precision by declining to answer hard questions and guessing on easier questions, despite not actually knowing how to deduce the answers of any of the questions. This points to the importance and difficulty of designing high-quality distractors, and to the importance of validating model performance on multiple-choice benchmarks by manual grading in an open-answer format.
In addition, the Cloning Scenarios are sufficiently laborious that even our human evaluators often did not find it worth their time to answer them, since they are much harder than the other questions in the benchmark. Thus, the human baseline presented here may be unreasonably low for trained molecular biologists. In designing “human-hard” benchmarks that are truly challenging for humans, we expect this to be an ongoing challenge, and the notion of a “human baseline” for harder tasks may cease to be meaningful.
Future Outlook:
We have released 80% of the LAB-Bench dataset today (available at HuggingFace and GitHub.) We are withholding a private test set of 20% that we will use to monitor for training contamination (models being overfit to the benchmark). Moreover, the process that we have developed internally for building these evals is scalable and extensible, and we expect to leverage it to create more challenging benchmarks like the Cloning Scenarios, as well as additional benchmarks addressing wet lab research and other areas of biology. If you are interested in joining us to work on evals, please get in touch at evals@futurehouse.org or see our open roles here. Likewise, if you have ideas for evaluations we should build or would like to work with us to leverage our pipeline to build specific evaluations, please get in touch at evals@futurehouse.org.
We are grateful to OpenPhilanthropy, whose generous support for our evaluation work made this work possible. In addition, we are grateful to Eric and Wendy Schmidt for supporting our research.
Access the paper on ArXiv: LINK
Download the pdf directly: LINK